Introduction
In the evolving landscape of artificial intelligence (AI) and machine learning, the importance of labeled data remains unabated. Labeled data is the basis for AI models to learn, predict and provide valuable insights. Nonetheless, the data annotation process, which often relies on human labeling, is not without challenges. These challenges include the large amounts of data required, the associated costs, and the potential for human bias that goes into labeled data.
Experience the transformative power of generative artificial intelligence. On this journey, we set out to explore generative AI models like SAM, DALL·E, and GPT, and their remarkable synergy in reimagining AI training. These models are at the forefront of data generation, annotation, and enhancement, providing innovative solutions to reduce reliance on human labelers while increasing the quality, efficiency, and ethical responsibility of AI development.
Understanding Generative AI
Generative AI is the bedrock of data generation and creativity in the AI landscape. It entails the creation of content—images, text, audio, and more—that often mirrors human-created data. Powered by advanced neural networks and pretrained on vast datasets, Generative AI models decode the intricate patterns, structures, and semantics of the data they generate.
Generative AI is not a single entity but rather a family of models, each with its own unique abilities. At its core, Generative AI is about learning and replicating patterns and information from existing data to create something new. These models operate on the principle of generative modeling, where they learn from data to generate content that exhibits similar patterns and characteristics.
SAM for Segmentation
The automation of complex data annotation tasks is at the heart of Generative AI's potential. Enter SAM, the Semantic Autoencoder for Multi-modal Data. SAM possesses a remarkable aptitude for automating segmentation tasks, a cornerstone of computer vision. By harnessing its understanding of multi-modal data, SAM can intelligently segment images, extract objects, and provide precise annotations, all without the need for extensive human intervention.
For example, in medical imaging, SAM can automatically delineate organs or abnormalities within images, allowing for faster and more accurate diagnoses. In autonomous driving, SAM can identify and segment various objects on the road, such as pedestrians, vehicles, and traffic signs, facilitating safer navigation.
DALL·E for Image Generation
In the realm of image generation, DALL·E emerges as an imaginative visionary. Developed by OpenAI, DALL·E has the power to transform textual descriptions into captivating visual representations. Fueled by a deep learning architecture pretrained on vast troves of textual and visual data, DALL·E opens new creative horizons, from artwork to advertising.
Consider a scenario where a user provides a textual description like "a surreal landscape with floating elephants and candy-colored clouds." DALL·E can take this description and generate an image that aligns perfectly with the user's imagination. This remarkable capability finds applications not only in creative endeavors but also in industries like design, where quick visualizations of concepts and ideas are invaluable.
DALL·E's and SAM's Contribution
In this scenario, DALL·E is responsible for creating the image. Based on a descriptive prompt, it generates a realistic and detailed image, like the one of the cats in the garden prompt was given to DALL-E plugin in Chatgpt 4. SAM would then analyze this image for segmentation. It identifies and delineates various elements within the image, such as the cat, individual plants, and other objects in the scene.
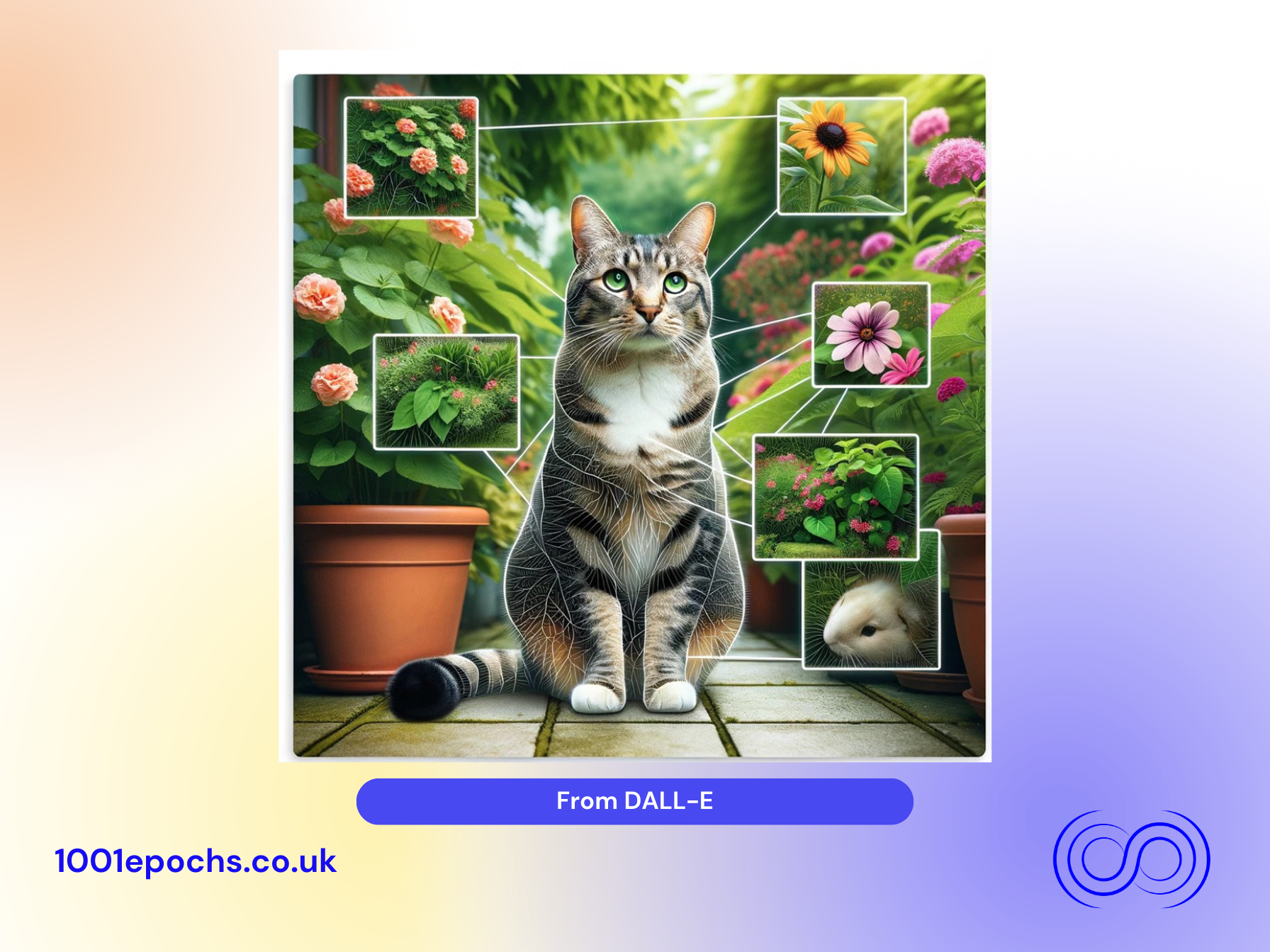
To represent SAM's segmentation which would be useful for labelling. For this we refer our audience to read more about SAM here and also to the official GitHub for installation on local system. For demo purposes their website to show the segmentation of the same object as shown in below figure. Here the tire is segmented of a vehicle and abounding box around the object can be seen.
.png)
GPT in Data Generation
Generative Pre-trained Transformers(GPT) are renowned for their natural language processing capabilities. However, GPT's versatility extends far beyond text generation. In the realm of data generation, GPT models contribute by producing textual descriptions annotations, and even synthetic data. This section explores how GPT models enhance data generation and complements SAM and DALL·E in the quest to reduce the reliance on human labelers.
Consider a scenario in which a company needs to generate textual product descriptions for thousands of items in its catalog. GPT can automate this process by taking basic information about each product and producing detailed and engaging descriptions, saving time and resources compared to manual writing.
Training AI Models with Synthetic Data
The foundation of AI training lies in labeled data. Synthetic data generation, powered by Generative AI, emerges as an enticing alternative. SAM, DALL·E, and GPT-generated data replicate real-world examples, reducing the need for extensive manual labeling. This section delves into the advantages, including enhanced training efficiency, cost savings, and potential for smaller, efficient models.
Let's consider an example in the field of robotics. Training a robot to recognize and manipulate objects in the real world requires a vast dataset of labeled images. Instead of manually labeling thousands of images of various objects, synthetic data generated by SAM and DALL·E can create a diverse training dataset with annotated objects, making the robot more capable and adaptable.
Ethical Considerations and Bias Mitigation
Generative AI integration into data generation and AI training raises ethical considerations. This section explores the implications, including bias amplification, transparency, accountability, privacy, and consent. It also outlines strategies for bias mitigation and responsible AI development.
Consider the potential pitfalls of using Generative AI to generate text for news articles. If not carefully monitored, the AI might inadvertently generate biased or misleading content. Ethical guidelines and oversight are essential to ensure that the AI-generated content aligns with journalistic standards and values.
The Future of AI Training
As we glimpse the future, Generative AI's transformative potential in AI training becomes clear. AI training efficiency will soar, ushering in smaller, specialized models. Ethical AI development will be standard, and data augmentation will reach new heights. This section explores promising advancements, including the intersection of Generative AI and explainability, as we navigate toward a future defined by innovation, efficiency, and ethical responsibility.
In the medical field, the combination off SAM-generated segmentation data, DALL·E-generated medical images, and GPT-generated patient case descriptions could revolutionize medical AI training. It could lead to the development of highly accurate diagnostic tools, improved patient care, and faster medical breakthroughs.
Conclusion
All in all, the journey from human labeling to generative AI-driven AI training represents an exciting chapter in the evolution of artificial intelligence. Generative AI models are reshaping the development of artificial intelligence with automatic data annotation, creative image generation and adaptive text synthesis. As we face such a future, the ethical imperative remains to ensure responsible AI development, transparency, and fairness. The promise of innovative and ethical A illuminates the path forward, where the potential for transformative impact is limitless. Generative AI is more than just a tool; It is a catalyst for future AI development to become easier, more efficient and more responsible.